Topological data analysis and its applications to machine learning
This course introduces the core concepts of topological data analysis (TDA), a set of methods from algebraic topology designed to study the structure of data. We will focus on key techniques such as persistence barcodes, which enable the uncovering of topological features in data that traditional methods fail to detect.
Throughout the course, we will explore how TDA can be applied to machine learning tasks, such as evaluating generative adversarial networks, dimensionality reduction, disentanglement, and detection of artificially generated texts. A solid understanding of the mathematical foundations behind these techniques will be developed, alongside practical experience in applying them to real-world problems.
The course will also touch on emerging research directions in TDA, including its integration with deep learning, the development of more efficient computational methods, and new applications in areas such as variational autoencoders and large language models.
Throughout the course, we will explore how TDA can be applied to machine learning tasks, such as evaluating generative adversarial networks, dimensionality reduction, disentanglement, and detection of artificially generated texts. A solid understanding of the mathematical foundations behind these techniques will be developed, alongside practical experience in applying them to real-world problems.
The course will also touch on emerging research directions in TDA, including its integration with deep learning, the development of more efficient computational methods, and new applications in areas such as variational autoencoders and large language models.
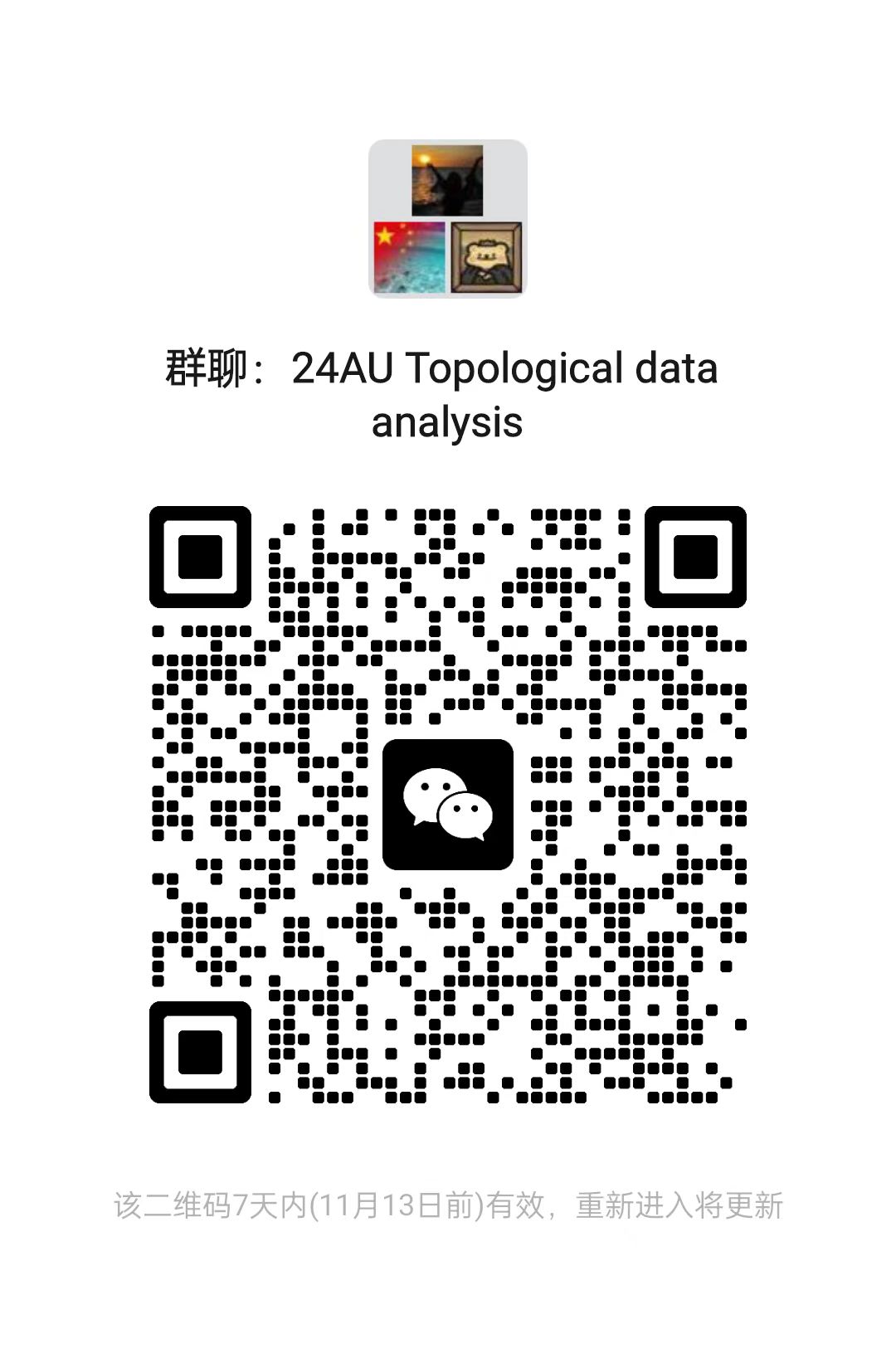
Lecturer
Date
11th November, 2024 ~ 15th January, 2025
Location
Weekday | Time | Venue | Online | ID | Password |
---|---|---|---|---|---|
Monday,Wednesday | 09:50 - 11:25 | A3-1a-205 | ZOOM 02 | 518 868 7656 | BIMSA |
Reference
[1] S. Barannikov, "The Framed Morse Complex and Its Invariants.", Advances in Soviet Mathematics, Volume 21, 1994, Pages 93–115, DOI: 10.1090/advsov/021/03
[2] S. Barannikov, "Canonical Forms = Persistence Diagrams", Tutorial. 37th European Workshop on Computational Geometry (EuroCG 2021)
[3] Le Peutrec, D., Nier, F. & Viterbo, C. “The Witten Laplacian and Morse–Barannikov Complex.” Ann. Henri Poincaré 14, 567–610 (2013).
[4] S. Barannikov, I. Trofimov, G. Sotnikov, E. Trimbach, A. Korotin, A. Filippov, E. Burnaev, "Manifold Topology Divergence: A Framework for Comparing Data Manifolds" Advances in Neural Information Processing Systems (NeurIPS 2021)
[5] S. Barannikov, I. Trofimov, N. Balabin, E. Burnaev, "Representation Topology Divergence: A Method for Comparing Neural Network Representations", 39th International Conference on Machine Learning (ICML 2022)
[2] S. Barannikov, "Canonical Forms = Persistence Diagrams", Tutorial. 37th European Workshop on Computational Geometry (EuroCG 2021)
[3] Le Peutrec, D., Nier, F. & Viterbo, C. “The Witten Laplacian and Morse–Barannikov Complex.” Ann. Henri Poincaré 14, 567–610 (2013).
[4] S. Barannikov, I. Trofimov, G. Sotnikov, E. Trimbach, A. Korotin, A. Filippov, E. Burnaev, "Manifold Topology Divergence: A Framework for Comparing Data Manifolds" Advances in Neural Information Processing Systems (NeurIPS 2021)
[5] S. Barannikov, I. Trofimov, N. Balabin, E. Burnaev, "Representation Topology Divergence: A Method for Comparing Neural Network Representations", 39th International Conference on Machine Learning (ICML 2022)
Video Public
Yes
Notes Public
Yes
Language
English
Lecturer Intro
Prof. Serguei Barannikov earned his Ph.D. from UC Berkeley and has made contributions to algebraic topology, algebraic geometry, mathematical physics, and machine learning. His work, prior to his Ph.D., introduced canonical forms of filtered complexes, now known as persistence barcodes, which have become fundamental in topological data analysis. More recently, he has applied topological methods to machine learning, particularly in the study of large language models, with results published in leading ML conferences such as NeurIPS, ICML, and ICLR, effectively bridging pure mathematics and advanced AI research.