Machine Learning, Neural Networks and Applications
This course is designed as an introduction to theoretical and practical aspects of machine learning, with a view to applications to problems in mathematics and sciences. It covers various foundational methods and best practices relevant to machine learning.
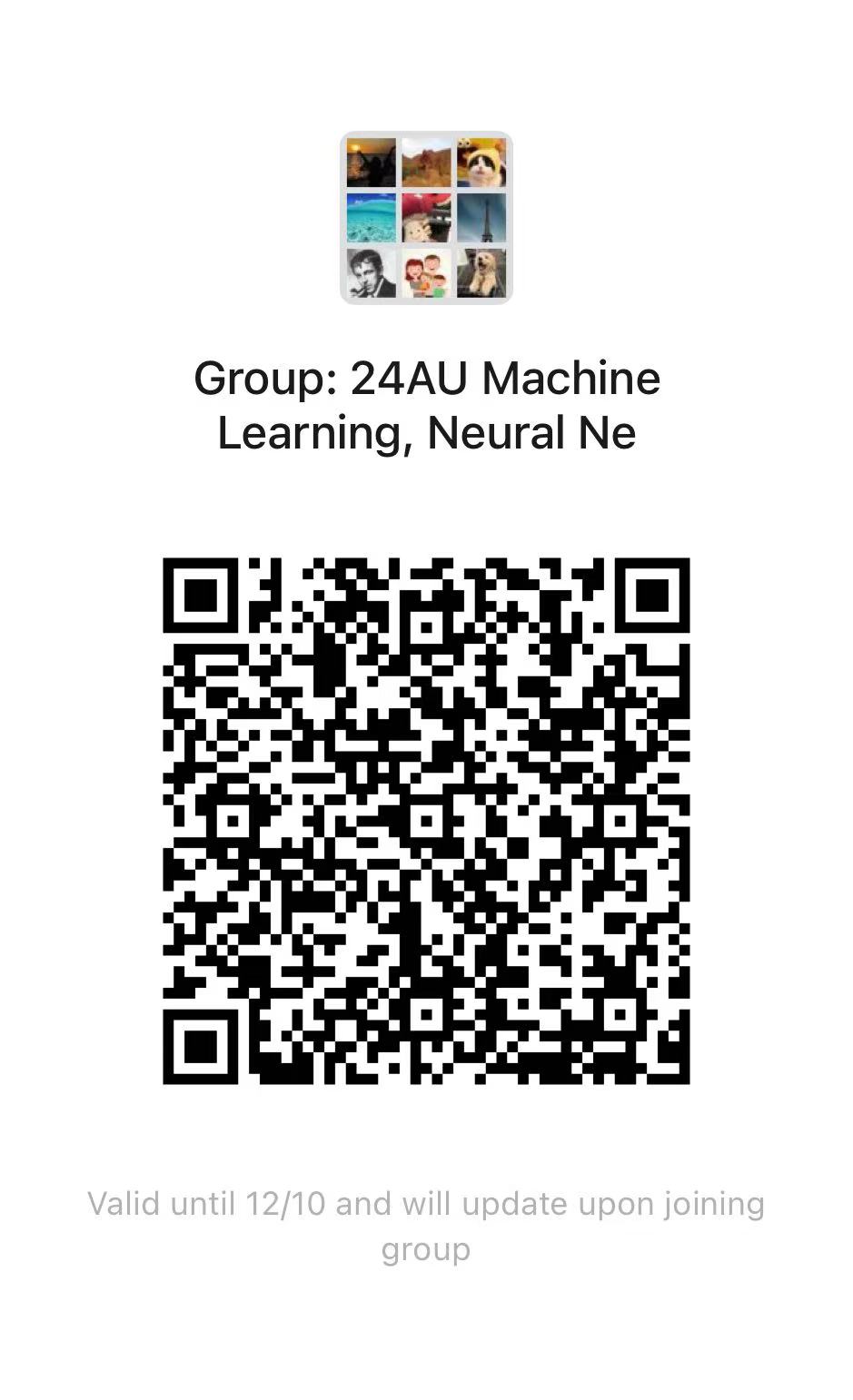
Lecturer
Date
18th September ~ 18th December, 2024
Location
Weekday | Time | Venue | Online | ID | Password |
---|---|---|---|---|---|
Tuesday,Wednesday | 09:50 - 11:25 | A3-1-103 | Zoom 15 | 204 323 0165 | BIMSA |
Prerequisite
Undergraduate Mathematics/Physics/Statistics
Syllabus
1. Introduction to Machine Learning: the Perceptron
2. Generalized Linear Models (Regression and Classification)
3. The Bias/Variance Tradeoff, Regularization
4. K-Nearest Neighbours and the curse of Dimensionality
5. Decision Trees, Bagging and Boosting
6. Support Vector Machines, Kernel Methods
7. Unsupervised Learning
8. Neural Networks: MLPs, Convolutional Networks, Sequence Models
9. Graph Neural Networks
2. Generalized Linear Models (Regression and Classification)
3. The Bias/Variance Tradeoff, Regularization
4. K-Nearest Neighbours and the curse of Dimensionality
5. Decision Trees, Bagging and Boosting
6. Support Vector Machines, Kernel Methods
7. Unsupervised Learning
8. Neural Networks: MLPs, Convolutional Networks, Sequence Models
9. Graph Neural Networks
Reference
Bishop: Pattern Recognition and Machine Learning
Goodfellow, Bengio, Courville: Deep Learning
Course Notes
Goodfellow, Bengio, Courville: Deep Learning
Course Notes
Audience
Undergraduate
, Advanced Undergraduate
, Graduate
Video Public
Yes
Notes Public
Yes
Language
English
Lecturer Intro
Dr Shailesh Lal received his PhD from the Harish-Chandra Research Institute. His research interests are applications of machine learning to string theory and mathematical physics, black holes in string theory and higher-spin holography.