Machine Learning, Neural Networks and Applications
This course is designed as an introduction to theoretical and practical aspects of machine learning, with a view to applications to problems in mathematics and sciences. It covers various foundational methods and best practices relevant to machine learning.
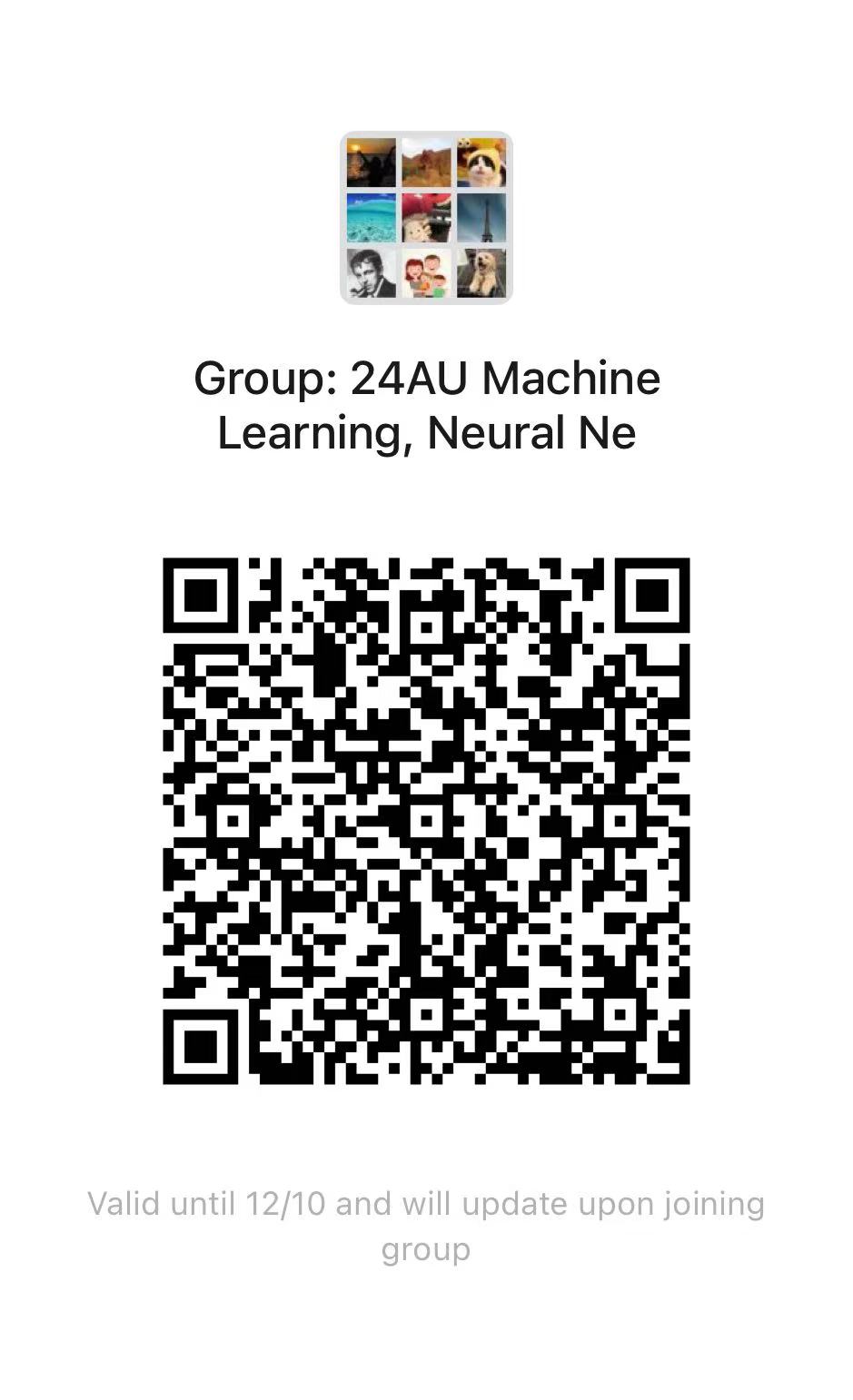
讲师
日期
2024年09月18日 至 12月18日
位置
Weekday | Time | Venue | Online | ID | Password |
---|---|---|---|---|---|
周二,周三 | 09:50 - 11:25 | A3-1-103 | Zoom 15 | 204 323 0165 | BIMSA |
修课要求
Undergraduate Mathematics/Physics/Statistics
课程大纲
1. Introduction to Machine Learning: the Perceptron
2. Generalized Linear Models (Regression and Classification)
3. The Bias/Variance Tradeoff, Regularization
4. K-Nearest Neighbours and the curse of Dimensionality
5. Decision Trees, Bagging and Boosting
6. Support Vector Machines, Kernel Methods
7. Unsupervised Learning
8. Neural Networks: MLPs, Convolutional Networks, Sequence Models
9. Graph Neural Networks
2. Generalized Linear Models (Regression and Classification)
3. The Bias/Variance Tradeoff, Regularization
4. K-Nearest Neighbours and the curse of Dimensionality
5. Decision Trees, Bagging and Boosting
6. Support Vector Machines, Kernel Methods
7. Unsupervised Learning
8. Neural Networks: MLPs, Convolutional Networks, Sequence Models
9. Graph Neural Networks
参考资料
Bishop: Pattern Recognition and Machine Learning
Goodfellow, Bengio, Courville: Deep Learning
Course Notes
Goodfellow, Bengio, Courville: Deep Learning
Course Notes
听众
Undergraduate
, Advanced Undergraduate
, Graduate
视频公开
公开
笔记公开
公开
语言
英文
讲师介绍
Shailesh Lal于Harish Chandra研究所获得博士学位。他的研究兴趣是机器学习在弦理论和数学物理中的应用,弦理论中的黑洞和higher-spin holography。