Deep Learning
Course Intruduction:
Master the fundamentals of deep learning and break into AI. Recently updated with cutting-edge techniques!
Deep Learning with code, math, and projects. Learning by doing!
Implemented with PyTorch, NumPy/MXNet, TensorFlow, Jax, and PaddlePaddle.
Build and train deep neural networks, identify key architecture parameters, implement vectorized neural networks and deep learning to applications
Train test sets, analyze variance for DL applications, use standard techniques and optimization algorithms, and build neural networks in PyTorch, etc.
Build a CNN and apply it to detection and recognition tasks, use neural style transfer to generate art, and apply algorithms to image and video data
Build and train RNNs, work with NLP and Word Embeddings, and use HuggingFace tokenizers and transformer models to perform NER and Question Answering
Skills you'll gain after complete the course:
Machine Learning expert
Recurrent Neural Network
PyTorch, NumPy/MXNet, TensorFlow, Jax, and PaddlePaddle
Convolutional Neural Network
Artificial Neural Network
Transformers
Course Complete Certification issued by BIMSA?
Master the fundamentals of deep learning and break into AI. Recently updated with cutting-edge techniques!
Deep Learning with code, math, and projects. Learning by doing!
Implemented with PyTorch, NumPy/MXNet, TensorFlow, Jax, and PaddlePaddle.
Build and train deep neural networks, identify key architecture parameters, implement vectorized neural networks and deep learning to applications
Train test sets, analyze variance for DL applications, use standard techniques and optimization algorithms, and build neural networks in PyTorch, etc.
Build a CNN and apply it to detection and recognition tasks, use neural style transfer to generate art, and apply algorithms to image and video data
Build and train RNNs, work with NLP and Word Embeddings, and use HuggingFace tokenizers and transformer models to perform NER and Question Answering
Skills you'll gain after complete the course:
Machine Learning expert
Recurrent Neural Network
PyTorch, NumPy/MXNet, TensorFlow, Jax, and PaddlePaddle
Convolutional Neural Network
Artificial Neural Network
Transformers
Course Complete Certification issued by BIMSA?
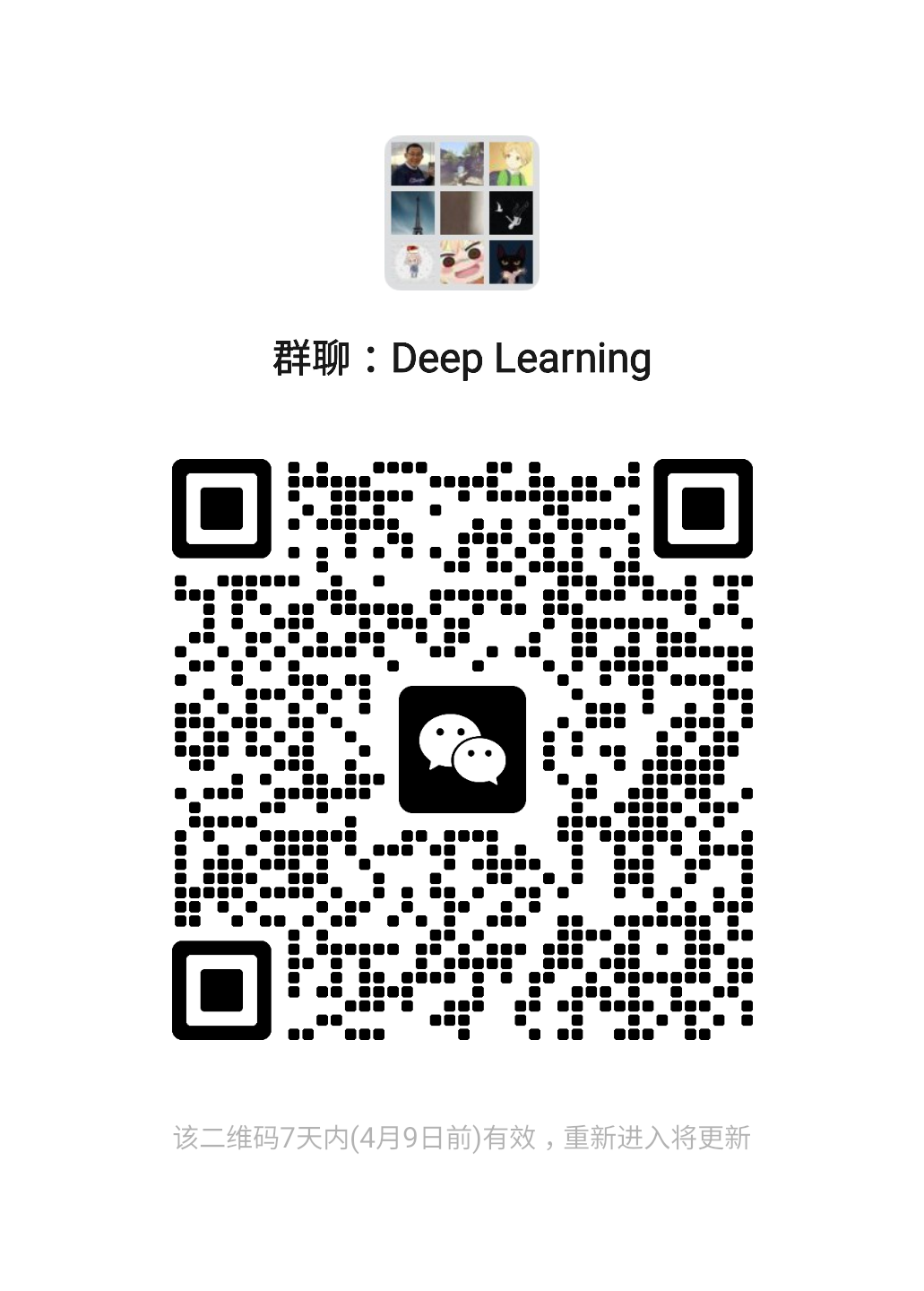
Lecturer
Date
8th April ~ 3rd July, 2024
Location
Weekday | Time | Venue | Online | ID | Password |
---|---|---|---|---|---|
Monday,Wednesday | 10:00 - 11:50 | A3-2a-201 | ZOOM 06 | 537 192 5549 | BIMSA |
Prerequisite
Algebra, Calculus, Probability, Statistics, Python
Syllabus
Preface/Syllabus/Installation
Lecture 1: Introduction
Lecture 2: Preliminaries
Lecture 3: Linear Neural Networks for Regression
Lecture 4: Linear Neural Networks for Classification
Lecture 5: Multilayer Perceptrons (MP)
Lecture 6: Builders’ Guide
Lecture 7: Convolutional Neural Networks (CNN)
Lecture 8: Modern Convolutional Neural Networks (AlexNet, VGG, NiN, GoogLeNet, ResNet, DenseNet)
Lecture 9: Recurrent Neural Networks (RNN)
Lecture 10: Modern Recurrent Neural Networks
Lecture 11: Attention Mechanisms and Transformers
Lecture 12: Optimization Algorithms
Lecture 13: Computational Performance
Lecture 14: Computer Vision
Lecture 15: Natural Language Processing: Pretraining (NLP)
Lecture 16: Natural Language Processing: Applications (NLP)
Lecture 17: Reinforcement Learning (RL)
Lecture 18: Gaussian Processes
Lecture 19: Hyperparameter Optimization
Lecture 20: Generative Adversarial Networks
Lecture 21: Recommender Systems
Lecture 1: Introduction
Lecture 2: Preliminaries
Lecture 3: Linear Neural Networks for Regression
Lecture 4: Linear Neural Networks for Classification
Lecture 5: Multilayer Perceptrons (MP)
Lecture 6: Builders’ Guide
Lecture 7: Convolutional Neural Networks (CNN)
Lecture 8: Modern Convolutional Neural Networks (AlexNet, VGG, NiN, GoogLeNet, ResNet, DenseNet)
Lecture 9: Recurrent Neural Networks (RNN)
Lecture 10: Modern Recurrent Neural Networks
Lecture 11: Attention Mechanisms and Transformers
Lecture 12: Optimization Algorithms
Lecture 13: Computational Performance
Lecture 14: Computer Vision
Lecture 15: Natural Language Processing: Pretraining (NLP)
Lecture 16: Natural Language Processing: Applications (NLP)
Lecture 17: Reinforcement Learning (RL)
Lecture 18: Gaussian Processes
Lecture 19: Hyperparameter Optimization
Lecture 20: Generative Adversarial Networks
Lecture 21: Recommender Systems
Reference
Material:
English Version https://d2l.ai/
Chinese Version https://zh.d2l.ai/
https://developers.google.com/machine-learning/
English Version https://d2l.ai/
Chinese Version https://zh.d2l.ai/
https://developers.google.com/machine-learning/
Audience
Graduate
, Postdoc
, Researcher
Video Public
Yes
Notes Public
Yes
Language
Chinese
, English
Lecturer Intro
After graduating with a PhD, he mainly worked in the field of wireless communications. He has worked in Lucent, Alcatel-Lucent, and Nokia as a senior engineer. He has 23 years of rich knowledge and experience in the field of wireless communications. Currently he is a research fellow of Beijing Institute of Mathematical Sciences and Applications (BIMSA) engaged in research on 4G/5G Wireless Communication, Biomathematics, Neural Network, Artificial Intelligence, Big Data, Machine Learning, nonlinear filter.