Deep Learning
Course Intruduction:
Master the fundamentals of deep learning and break into AI. Recently updated with cutting-edge techniques!
Deep Learning with code, math, and projects. Learning by doing!
Implemented with PyTorch, NumPy/MXNet, TensorFlow, Jax, and PaddlePaddle.
Build and train deep neural networks, identify key architecture parameters, implement vectorized neural networks and deep learning to applications
Train test sets, analyze variance for DL applications, use standard techniques and optimization algorithms, and build neural networks in PyTorch, etc.
Build a CNN and apply it to detection and recognition tasks, use neural style transfer to generate art, and apply algorithms to image and video data
Build and train RNNs, work with NLP and Word Embeddings, and use HuggingFace tokenizers and transformer models to perform NER and Question Answering
Skills you'll gain after complete the course:
Machine Learning expert
Recurrent Neural Network
PyTorch, NumPy/MXNet, TensorFlow, Jax, and PaddlePaddle
Convolutional Neural Network
Artificial Neural Network
Transformers
Course Complete Certification issued by BIMSA?
Master the fundamentals of deep learning and break into AI. Recently updated with cutting-edge techniques!
Deep Learning with code, math, and projects. Learning by doing!
Implemented with PyTorch, NumPy/MXNet, TensorFlow, Jax, and PaddlePaddle.
Build and train deep neural networks, identify key architecture parameters, implement vectorized neural networks and deep learning to applications
Train test sets, analyze variance for DL applications, use standard techniques and optimization algorithms, and build neural networks in PyTorch, etc.
Build a CNN and apply it to detection and recognition tasks, use neural style transfer to generate art, and apply algorithms to image and video data
Build and train RNNs, work with NLP and Word Embeddings, and use HuggingFace tokenizers and transformer models to perform NER and Question Answering
Skills you'll gain after complete the course:
Machine Learning expert
Recurrent Neural Network
PyTorch, NumPy/MXNet, TensorFlow, Jax, and PaddlePaddle
Convolutional Neural Network
Artificial Neural Network
Transformers
Course Complete Certification issued by BIMSA?
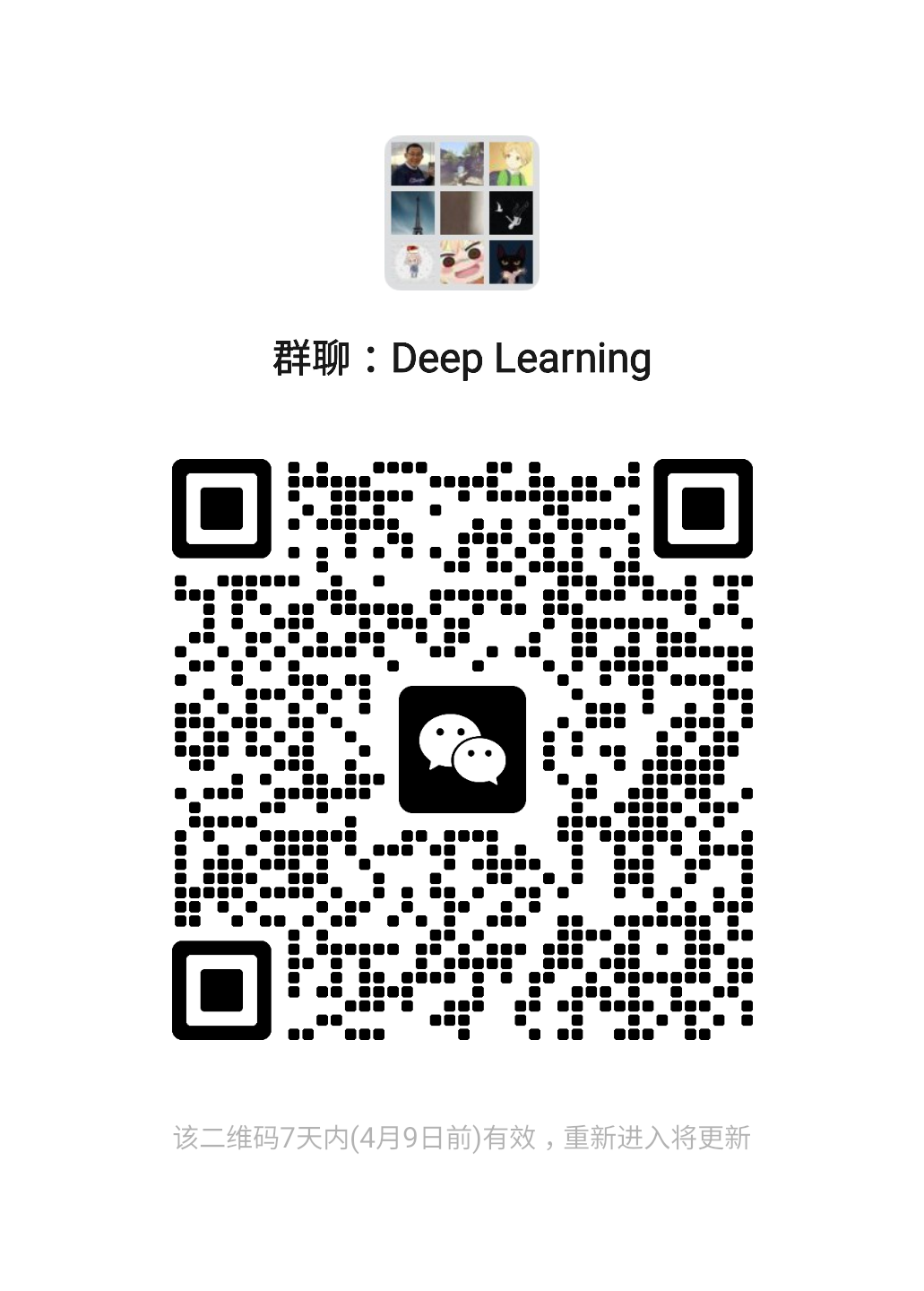
讲师
日期
2024年04月08日 至 07月03日
位置
Weekday | Time | Venue | Online | ID | Password |
---|---|---|---|---|---|
周一,周三 | 10:00 - 11:50 | A3-2a-201 | ZOOM 06 | 537 192 5549 | BIMSA |
修课要求
Algebra, Calculus, Probability, Statistics, Python
课程大纲
Preface/Syllabus/Installation
Lecture 1: Introduction
Lecture 2: Preliminaries
Lecture 3: Linear Neural Networks for Regression
Lecture 4: Linear Neural Networks for Classification
Lecture 5: Multilayer Perceptrons (MP)
Lecture 6: Builders’ Guide
Lecture 7: Convolutional Neural Networks (CNN)
Lecture 8: Modern Convolutional Neural Networks (AlexNet, VGG, NiN, GoogLeNet, ResNet, DenseNet)
Lecture 9: Recurrent Neural Networks (RNN)
Lecture 10: Modern Recurrent Neural Networks
Lecture 11: Attention Mechanisms and Transformers
Lecture 12: Optimization Algorithms
Lecture 13: Computational Performance
Lecture 14: Computer Vision
Lecture 15: Natural Language Processing: Pretraining (NLP)
Lecture 16: Natural Language Processing: Applications (NLP)
Lecture 17: Reinforcement Learning (RL)
Lecture 18: Gaussian Processes
Lecture 19: Hyperparameter Optimization
Lecture 20: Generative Adversarial Networks
Lecture 21: Recommender Systems
Lecture 1: Introduction
Lecture 2: Preliminaries
Lecture 3: Linear Neural Networks for Regression
Lecture 4: Linear Neural Networks for Classification
Lecture 5: Multilayer Perceptrons (MP)
Lecture 6: Builders’ Guide
Lecture 7: Convolutional Neural Networks (CNN)
Lecture 8: Modern Convolutional Neural Networks (AlexNet, VGG, NiN, GoogLeNet, ResNet, DenseNet)
Lecture 9: Recurrent Neural Networks (RNN)
Lecture 10: Modern Recurrent Neural Networks
Lecture 11: Attention Mechanisms and Transformers
Lecture 12: Optimization Algorithms
Lecture 13: Computational Performance
Lecture 14: Computer Vision
Lecture 15: Natural Language Processing: Pretraining (NLP)
Lecture 16: Natural Language Processing: Applications (NLP)
Lecture 17: Reinforcement Learning (RL)
Lecture 18: Gaussian Processes
Lecture 19: Hyperparameter Optimization
Lecture 20: Generative Adversarial Networks
Lecture 21: Recommender Systems
参考资料
Material:
English Version https://d2l.ai/
Chinese Version https://zh.d2l.ai/
https://developers.google.com/machine-learning/
English Version https://d2l.ai/
Chinese Version https://zh.d2l.ai/
https://developers.google.com/machine-learning/
听众
Graduate
, 博士后
, Researcher
视频公开
公开
笔记公开
公开
语言
中文
, 英文
讲师介绍
博士毕业后主要从事无线通信领域方面的工作,先后在朗讯,阿尔卡特-朗讯,诺基亚公司任职,资深工程师,具有23年无线通讯领域的丰富知识和经验。目前就职于北京雁栖湖应用数学研究院的研究员从事生物数学,人工智能,神经网络,机器学习,大数据,非线性滤波方面的研究。