Data-Driven Science and Engineering
This course teaches from the book 《Data-Driven Science and Engineering》 by Prof. Steven L. Brunton and Prof. J. Nathan Kutz. This book brings together machine learning, engineering mathematics, and mathematical physics to integrate modeling and control of dynamical systems with modern methods in data science. It highlights many of the recent advances in scientific computing that enable data-driven methods to be applied to a diverse range of complex systems, such as turbulence, the brain, climate, epidemiology, finance, robotics, and autonomy. Aimed at advanced undergraduate and beginning graduate students in the engineering and physical sciences, the text presents a range of topics and methods from introductory to state of the art.
本课程采用由Steven L. Brunton教授和J. Nathan Kutz教授编写的《Data-Driven Science and Engineering》一书进行教学。该书结合了机器学习、工程数学和数学物理,将动力系统的建模和控制与现代数据科学方法整合在一起。它强调了科学计算中许多最新的进展,这些进展使得数据驱动的方法可以应用于各种复杂系统,如湍流、大脑、气候、流行病学、金融、机器人和自主系统等。该课程面向高年级本科生和低年级研究生,涵盖了从入门到前沿的各类主题和方法。
本课程采用由Steven L. Brunton教授和J. Nathan Kutz教授编写的《Data-Driven Science and Engineering》一书进行教学。该书结合了机器学习、工程数学和数学物理,将动力系统的建模和控制与现代数据科学方法整合在一起。它强调了科学计算中许多最新的进展,这些进展使得数据驱动的方法可以应用于各种复杂系统,如湍流、大脑、气候、流行病学、金融、机器人和自主系统等。该课程面向高年级本科生和低年级研究生,涵盖了从入门到前沿的各类主题和方法。
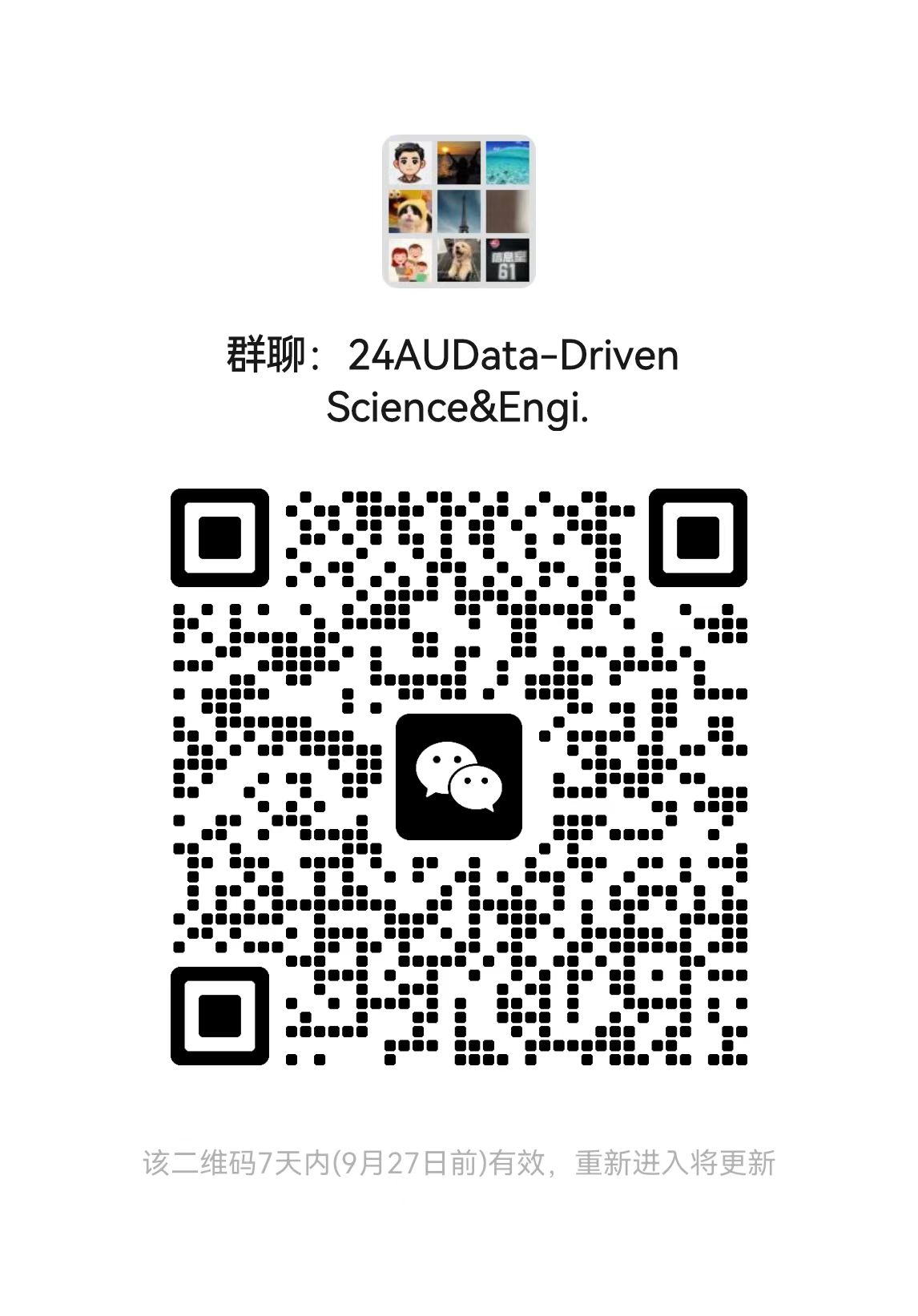
Lecturer
Date
13th September, 2024 ~ 3rd January, 2025
Location
Weekday | Time | Venue | Online | ID | Password |
---|---|---|---|---|---|
Friday | 14:20 - 16:55 | A3-4-101 | ZOOM 01 | 928 682 9093 | BIMSA |
Prerequisite
optimization, matrix computation, statistics, differential equation, python
Syllabus
I Dimensionality Reduction and Transforms 1
1 Singular Value Decomposition (SVD)
2 Fourier and Wavelet Transforms
3 Sparsity and Compressed Sensing
II Machine Learning and Data Analysis
4 Regression and Model Selection
5 Clustering and Classification
6 Neural Networks and Deep Learning
III Dynamics and Control
7 Data-Driven Dynamical Systems
8 Linear Control Theory
9 Balanced Models for Control
IV Advanced Data-Driven Modeling and Control
10 Data-Driven Control
11 Reinforcement Learning
12 Reduced-Order Models (ROMs)
13 Interpolation for Parametric Reduced-Order Models
14 Physics-Informed Machine Learning
1 Singular Value Decomposition (SVD)
2 Fourier and Wavelet Transforms
3 Sparsity and Compressed Sensing
II Machine Learning and Data Analysis
4 Regression and Model Selection
5 Clustering and Classification
6 Neural Networks and Deep Learning
III Dynamics and Control
7 Data-Driven Dynamical Systems
8 Linear Control Theory
9 Balanced Models for Control
IV Advanced Data-Driven Modeling and Control
10 Data-Driven Control
11 Reinforcement Learning
12 Reduced-Order Models (ROMs)
13 Interpolation for Parametric Reduced-Order Models
14 Physics-Informed Machine Learning
Reference
http://www.databookuw.com
Audience
Advanced Undergraduate
, Graduate
, Postdoc
, Researcher
Video Public
Yes
Notes Public
No
Language
Chinese
Lecturer Intro
Yunfeng Cai studied in Mathematics at the University of Science and Technology of China from 2000 to 2004. He then pursued his PhD in Computing Mathematics at Peking University, which he obtained in Jan. 2009. From Jan. 2009 to June 2012, he worked as a postdoctoral researcher at the Academy of Mathematics and Systems Science / University of California, Davis. From Sep. 2012 to Sep. 2018, he served as a researcher at Peking University. In Sep. 2018, he joined Baidu Research as a Research Scientist and left on May 2024. In June 2024, he began his current role as a Professor at the Beijing Institute of Mathematical Sciences and Applications (BIMSA).