AI for Solving Forward and Inverse PDE Problems
AI for Science is currently a research hotspot. This series of courses continues to introduce machine learning (ML) methods for solving forward and inverse problems of partial differential equations. Compared to previous courses, this semester's course places more emphasis on using lecture notes summarized and written by the instructor. Meanwhile, for each knowledge point of ML in modelling and scientific computing, the latest published literatures will be supplemented for explanation, including methods, numerical examples, and codes. Audiences studying on the relevant topics are encouraged to present your research and interact more.
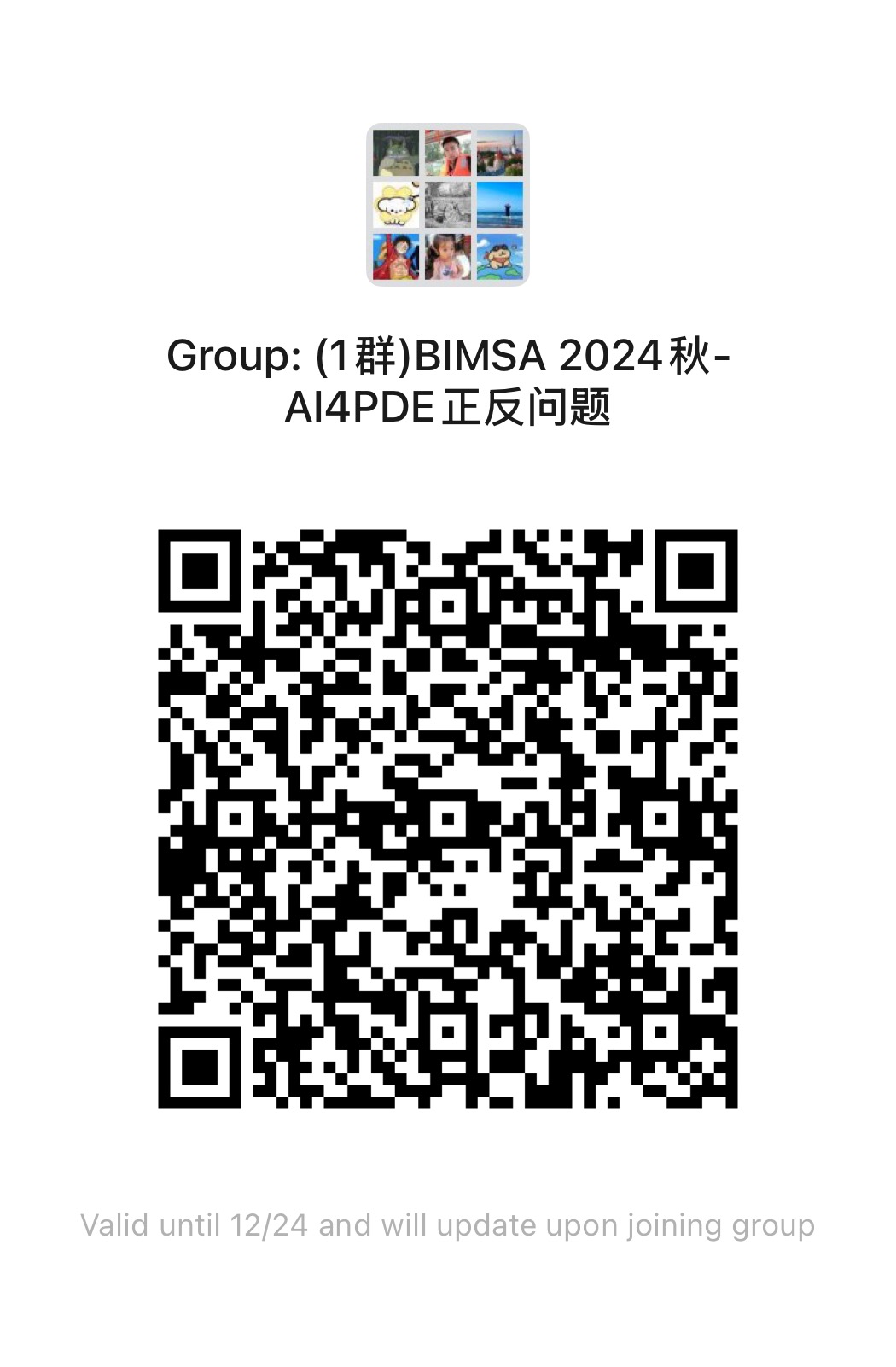
Lecturer
Date
8th October ~ 24th December, 2024
Location
Weekday | Time | Venue | Online | ID | Password |
---|---|---|---|---|---|
Tuesday | 13:30 - 16:55 | A3-1-301 | ZOOM 06 | 537 192 5549 | BIMSA |
Prerequisite
Basic knowledges on deep neural networks, partial differential equations, and the Python language.
Syllabus
1. Introduction to important knowledge points related to Machine Learning and Differential Equations, Some review literatures
ML Methods for Solving Forward Problems:
2. Physics-Informed Neural Networks (a)
3. Physics-Informed Neural Networks (b)
4. Physics-Informed Neural Networks (c)
ML Methods for Solving Inverse Problems:
5. Neural Network Surrogate Modeling Method
6. Discovery of ODE/PDE from Data (a)
7. Discovery of ODE/PDE from Data (b)
Operator Learning & Reduced Order Modeling:
8. Deep Neural Operator (a)
9. Deep Neural Operator (b)
10. Reduced Order Modeling (a)
11. Reduced Order Modeling (b)
12. Course review, Communication, and Interaction
ML Methods for Solving Forward Problems:
2. Physics-Informed Neural Networks (a)
3. Physics-Informed Neural Networks (b)
4. Physics-Informed Neural Networks (c)
ML Methods for Solving Inverse Problems:
5. Neural Network Surrogate Modeling Method
6. Discovery of ODE/PDE from Data (a)
7. Discovery of ODE/PDE from Data (b)
Operator Learning & Reduced Order Modeling:
8. Deep Neural Operator (a)
9. Deep Neural Operator (b)
10. Reduced Order Modeling (a)
11. Reduced Order Modeling (b)
12. Course review, Communication, and Interaction
Reference
1. The lecture notes written by the instructor.
2. Latest published literatures related to machine learning and differential equations, which will be recommended before each class.
2. Latest published literatures related to machine learning and differential equations, which will be recommended before each class.
Audience
Undergraduate
, Advanced Undergraduate
, Graduate
, Postdoc
, Researcher
Video Public
No
Notes Public
No
Language
Chinese
Lecturer Intro
Fansheng Xiong (熊繁升) is currently an Assistant Researcher Fellow of BIMSA. Before that, he got a bachelor's degree from China University of Geosciences (Beijing), and a doctoral degree from Tsinghua University. He was a visiting student at Yale University for one year. His research interest mainly focuses on solving PDE-related forward/inverse problems based on machine learning algorithms (DNN, PINN, DeepONet, etc.), and their applications in geophysical wave propagation problems and turbulence modeling of fluid mechanics. The relevant efforts have been published in journals such as JGR Solid Earth, GJI, Geophysics, etc.