Safety of Large Language Models
This course introduces students to the core principles and challenges surrounding large-scale neural language models' safe and responsible development. It is designed for graduate students and technical professionals with prior experience in machine learning and natural language processing.
The course will explore the basics of LLMs, including architectural foundations, training procedures. The second part of the course goes deeper with exploring vulnerabilities such as hallucinations and adversarial attacks, and recent advances in aligning LLMs with human intent and values.
List of Lectures
1. Introduction to Transformer Models and LLMs
2. Training of LLMs: From Pretraining to Fine-tuning
3. Hallucination Detection in LLMs
4. Adversarial Attacks on Language Models
5. Alternatives to Transformers: LLMs and State-space models
The course will explore the basics of LLMs, including architectural foundations, training procedures. The second part of the course goes deeper with exploring vulnerabilities such as hallucinations and adversarial attacks, and recent advances in aligning LLMs with human intent and values.
List of Lectures
1. Introduction to Transformer Models and LLMs
2. Training of LLMs: From Pretraining to Fine-tuning
3. Hallucination Detection in LLMs
4. Adversarial Attacks on Language Models
5. Alternatives to Transformers: LLMs and State-space models
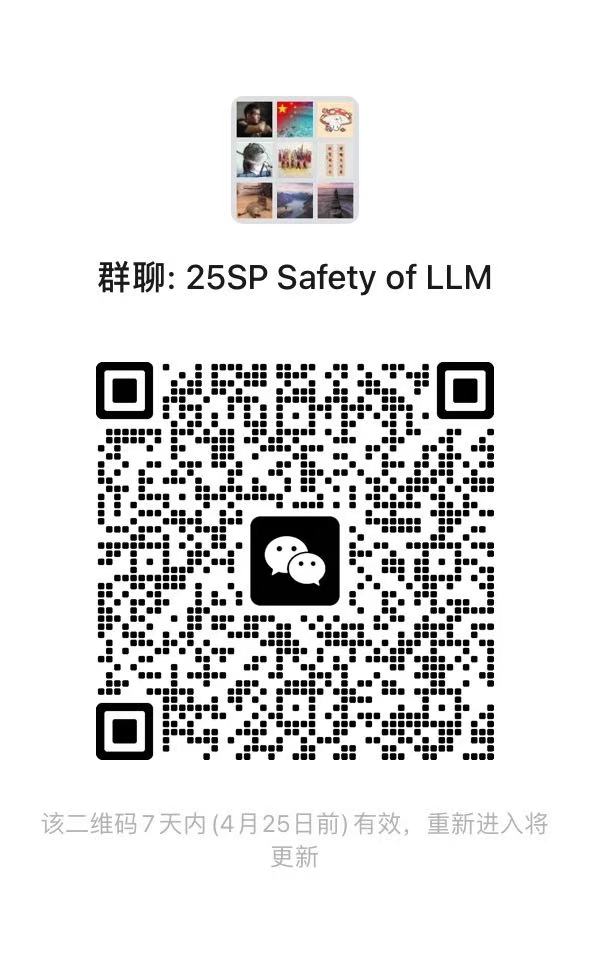
讲师
Alexey Zaytsev
日期
2025年04月16日 至 25日
位置
Weekday | Time | Venue | Online | ID | Password |
---|---|---|---|---|---|
周一,周三,周五 | 09:50 - 12:15 | A3-4-312 | ZOOM 12 | 815 762 8413 | BIMSA |
课程大纲
Lecture 1 – Introduction to Transformer Models and LLMs
● Overview of the Transformer architecture: attention mechanism, positional encoding, and multi-head attention
● Decoder-only vs encoder-decoder vs encoder-only configurations
● Key developments in LLMs: GPT series, BERT, T5, LLaMA, PaLM
● Scaling laws: impact of model size, dataset size, and compute
● Architectural choices relevant to safety (e.g., sparse attention, Mixture of Experts)
Lecture 2 – Training of LLMs: From Pretraining to Fine-tuning
● Optimisation problems behind the training of LLMs
● Stages of training:
○ Pretraining: objectives with causal language and masked language modeling
○ Supervised fine-tuning: instruction-following datasets
○ Low-rank updates
● Differences between open-ended generation and task-oriented tuning
● Where and how safety issues arise during training (e.g., data contamination, overfitting, misalignment)
Lecture 3 – Hallucination Detection in LLMs
● Definition and taxonomy of hallucinations: factual, logical, extractive
● Causes of hallucinations in LLMs: overgeneralisation, data gaps, lack of grounding
● Methods for detection and evaluation:
○ Factual verification against knowledge bases
○ Self-consistency and uncertainty estimation
○ Retrieval-augmented methods
Lecture 4 – Adversarial Attacks on Language Models
● Introduction to adversarial attacks
● Challenges for adversarial attacks in NLP
● Gradient-based attacks
● Universal adversarial triggers and fine-tuning vulnerabilities
● Poisoning attacks
● Defence strategies and model hardening
Lecture 5 – Alignment of LLMs
● The alignment problem: mismatches between learned behaviour and human intent
● Common approaches:
○ Supervised fine-tuning with curated data
○ Reinforcement Learning from Human Feedback (RLHF)
○ Constitutional AI: rule-based alignment without human labels
● Tradeoffs between helpfulness, harmlessness, and honesty
● Limitations of current methods and ongoing research directions in scalable oversight and interpretability
● Overview of the Transformer architecture: attention mechanism, positional encoding, and multi-head attention
● Decoder-only vs encoder-decoder vs encoder-only configurations
● Key developments in LLMs: GPT series, BERT, T5, LLaMA, PaLM
● Scaling laws: impact of model size, dataset size, and compute
● Architectural choices relevant to safety (e.g., sparse attention, Mixture of Experts)
Lecture 2 – Training of LLMs: From Pretraining to Fine-tuning
● Optimisation problems behind the training of LLMs
● Stages of training:
○ Pretraining: objectives with causal language and masked language modeling
○ Supervised fine-tuning: instruction-following datasets
○ Low-rank updates
● Differences between open-ended generation and task-oriented tuning
● Where and how safety issues arise during training (e.g., data contamination, overfitting, misalignment)
Lecture 3 – Hallucination Detection in LLMs
● Definition and taxonomy of hallucinations: factual, logical, extractive
● Causes of hallucinations in LLMs: overgeneralisation, data gaps, lack of grounding
● Methods for detection and evaluation:
○ Factual verification against knowledge bases
○ Self-consistency and uncertainty estimation
○ Retrieval-augmented methods
Lecture 4 – Adversarial Attacks on Language Models
● Introduction to adversarial attacks
● Challenges for adversarial attacks in NLP
● Gradient-based attacks
● Universal adversarial triggers and fine-tuning vulnerabilities
● Poisoning attacks
● Defence strategies and model hardening
Lecture 5 – Alignment of LLMs
● The alignment problem: mismatches between learned behaviour and human intent
● Common approaches:
○ Supervised fine-tuning with curated data
○ Reinforcement Learning from Human Feedback (RLHF)
○ Constitutional AI: rule-based alignment without human labels
● Tradeoffs between helpfulness, harmlessness, and honesty
● Limitations of current methods and ongoing research directions in scalable oversight and interpretability
视频公开
公开
笔记公开
公开
语言
英文
讲师介绍
Alexey has deep expertise in machine learning and processing of sequential data. He publishes at top venues, including KDD, ACM Multimedia and AISTATS. Industrial applications of his results are now in service at companies Airbus, Porsche and Saudi Aramco among others.