Mathematical Theory in Deep Learning
This course explores the fundamental mathematical theories underpinning deep learning. It covers the theoretical analysis of neural networks' approximation capabilities through universal approximation theorems, examines optimization models in the training process including loss landscape analysis and convergence properties, and investigates mathematical frameworks for generalization including statistical learning theory and overparameterization phenomena. The course also addresses the mathematical foundations of generative models, analyzing theories behind VAEs, GANs, and diffusion models through optimal transport and manifold learning perspectives.
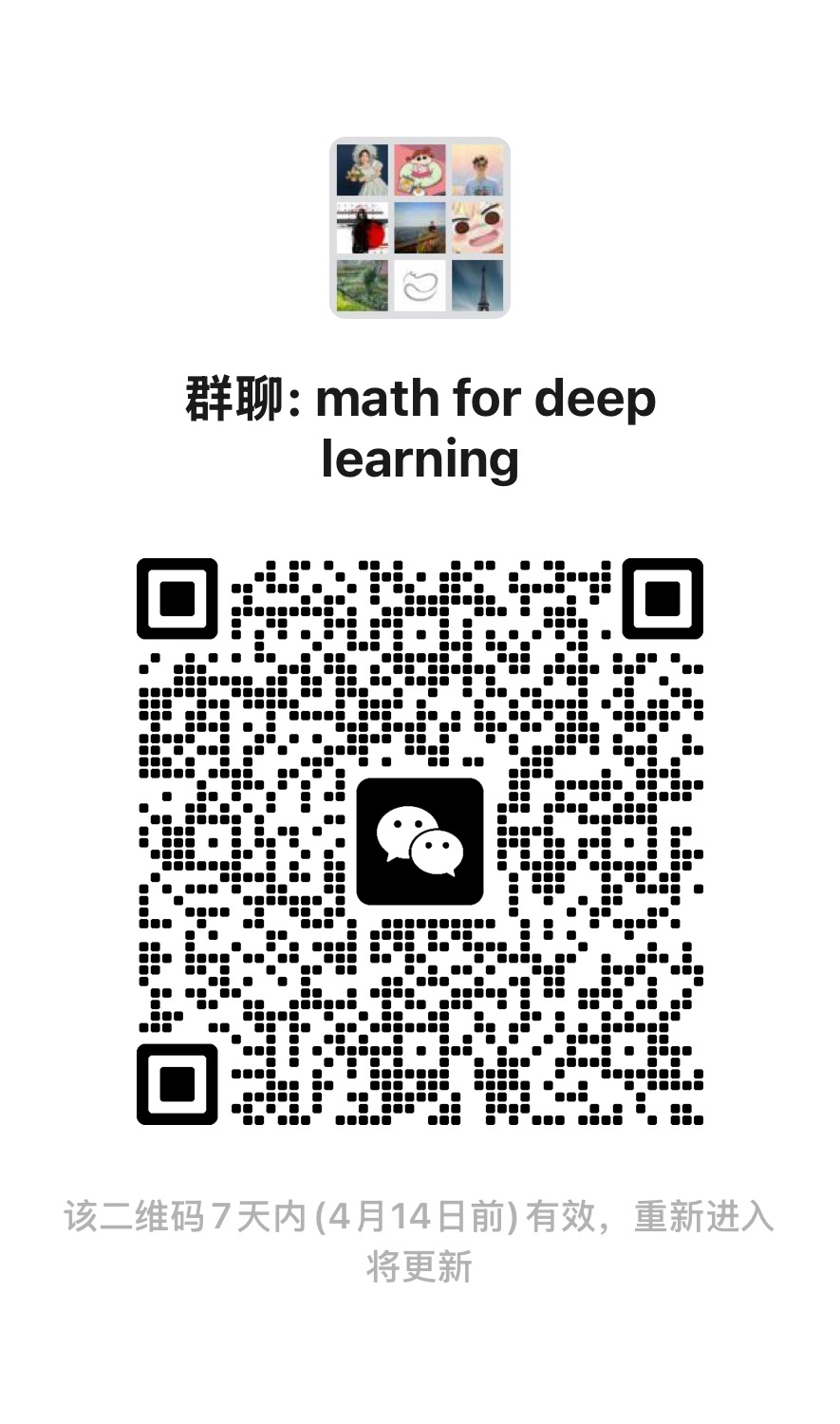
讲师
日期
2025年02月25日 至 06月10日
位置
Weekday | Time | Venue | Online | ID | Password |
---|---|---|---|---|---|
周二 | 13:30 - 16:05 | A3-4-101 | ZOOM 13 | 637 734 0280 | BIMSA |
听众
Advanced Undergraduate
, Graduate
视频公开
公开
笔记公开
公开
语言
英文